Abstract
The ability to access and process massive amounts of online information is required in many learning models and situations. Research has repeatedly shown, however, that students do not spontaneously develop full-fletched online search skills and thus are unable to successfully solve problems that require web search. In order to develop a better understanding of student online search process especially in academic contexts, we developed a research tool, SCOOP, which aids researchers to gather and analyze relevant information to detect this process. This study involved 12 male students in a Chinese university. All of them were familiar with computers and web. We recorded their search activities with SCOOP as they searched for answers to two essay questions. We evaluated the data by redrawing the search path for each participant and then visually comparing the patterns across participants. We characterized the participants into four types of searcher – “active regulatory searchers”, “inactive regulatory searchers”, “non-regulatory searchers”, and “incapable searchers”. SCOOP builds a more extensive account of student web search process by tracking mouse behavior on web. It is a promising tool that researchers can use to collect online information on web search.
Keywords: Multiage instruction, non-graded classroom
Introduction
The Internet has become a ubiquitous information source in our daily life. Students are often given assignments that require them to search for information, including writing essays on certain topics, a biology report, or a student teacher’s analysis of questioning techniques in class. Despite a variety of sources to search for information with a particular purpose (e.g., in the library or with an encyclopedia), the most probable source a student would use nowadays is the World Wide Web (WWW). The ability to access and process massive amounts of information is required in many learning models and situations. This generic skill, however, presents both benefits and challenges to learners. Research has repeatedly shown that students do not spontaneously develop full- fletched online search skills and thus are unable to successfully solve problems that require web search (e.g., Hirsch, 1999; MaKinster, Beghetto, & Plucker, 2002; Monereo, Fuentes, & Sànchez, 2000). In order to develop a better understanding of student online search process especially in academic contexts, we developed a research tool which aids researchers to gather and analyze relevant information to detect the difficulties students are faced with while searching for information to accomplish academic tasks.
Related Work
A variety of web-tracking tools have been developed to trace user behaviour on the Web. For example, WebTracker (Turnbull, 2006) records menu choices, button bar selections, and keystroke actions, all tagged with a date-time stamp. Other similar tools available for logging user data in browsers include Cheese (Mueller & Lockerd, 2001), The Wrapper (Jansen, 2005), WebQuilt (2001) and WebLogger (Reeder, Pirolli, & Card, 2000). Some of them record more than mouse movement, for example, WebLogger can also capture scrolling a webpage and save the actual Web content (i.e. the text, images, scripts, etc.) at which a user looked during a browsing session (also see Goecks & Shavlik, 2000). More recently, MouseTracker (Freeman & Ambady, 2010) steps further by recording the streaming x-, y-coordinates of the computer mouse while users move the mouse into one of multiple response alternatives, and motor dynamics of the hand reveal the time course of mental processes.
Regardless the level of sophistication of these tools, the user-internet interaction has been mainly restricted to webpage access alone. While these tracking methods allow researchers to reconstruct move-by-move how individuals look for and access information on the Web, how they evaluate and select information with high relevance and quality within the sources is also critical, as it determines the effectiveness and efficiency of online search. This strategy facilitates the search for information and is adapted in case inadequate information is located within the selected sources. Hence, we also attend to this aspect while designing the tracking tool by offering users the opportunity to highlight relevant information in webpages during the search. In addition, our system provides the means to find out exactly how individuals search for information for a given task, and thus affords an extensive user model. As these log data are analyzed post hoc, search behaviour trends could be identified. This paper goes through the initial investigation of this technique and illustrates with real user data how the system can be utilized in behavioural, cognitive, and metacognitive research.
SCOOP
Tool Description
SCOOP is a web-browser add-on application developed for Firefox web-browser that detects and records significant events during a session of web search. After installation, it is displayed in a separate tool bar of the browser. When a user signs in with an assigned ID, the application is launched and runs without obtrusive interference with user web search. SCOOP documents user actions at five levels – time of an action, action name, object of action, details of object, and information output. The time of an action specifies the time point (with one-second precision) when an action starts. The action name indicates user actions on the mouse or the keyboard, such as clicks, key presses or menu selections to highlight information. The object of action defines the nature of actions users perform on. This can be the search button the user hits, the window he opens, the highlight he creates, or the hyperlink he clicks. The details of object corresponds to action-specific parameters, such as search query entered by the user, name of the window being accessed, the complete URL clicked, and the information highlighted. The information output denotes the actual URL of each information source. As seen in Figure 1, all events are recorded in a format corresponding to the aforementioned five levels, and saved in EXCEL files. They are ready for both humans and analysis software to read, process, aggregate and analyze.
Log Files and Log Analysis Tool
All the data collected in real time are sent to the server and available for instant viewing after a study session is ended. Researchers can search for logs based on a particular user ID or a particular date. All the logs that belong to that user or the specified date will be shown and can be downloaded easily (Figure 2). Conventional data analyses can be conducted for descriptive statistics (e.g., frequency counts) of an action, or inferential statistical analysis with popular software packages (e.g., EXCEL, SPSS). For pattern-based analyses, we developed a separate analysis tool, which can parse and mine the logs into patterns after clustering students by a pre-defined standard, such as task performance level, gender, learning styles, and etc. To prepare for parsing a log file, the researcher creates an action library that defines each multi-event action in terms of the fine-grained events in the log. For example, a task-definition action can be defined to include the following events: (a) click on the writing window to view the task question, (b) switch to the search window to search, and (c) input a search query to complete the search. When the above three steps are repeated, we can infer that the student was trying to monitor the search query choice to solve the task. The identified events in a log file are matched to the canonical action patterns defined by the researcher in the action library and the log is translated into an action file consisting of temporally ordered learner actions.
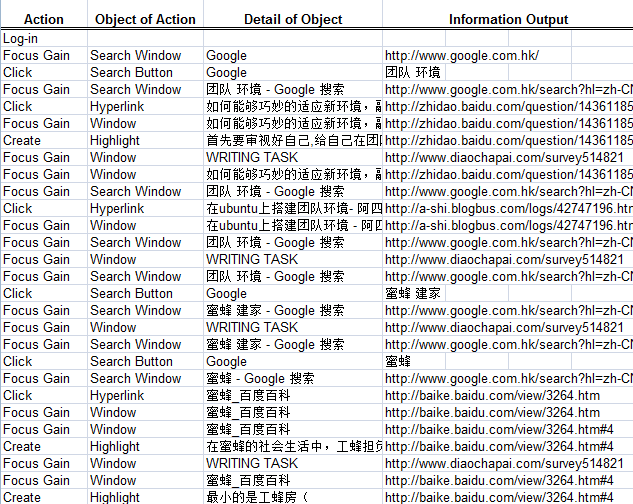
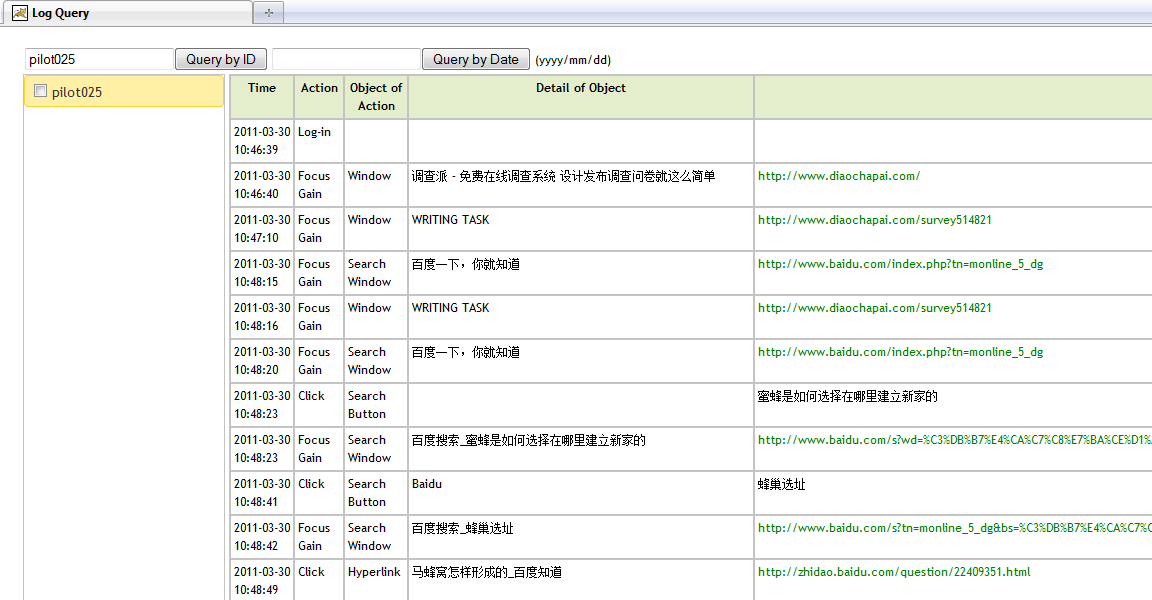
User Study
To evaluate the effectiveness and efficiency of SCOOP, we conducted a user study to uncover behavior trends in web search. This study involved 12 male students in a Chinese university. On average, the participants spent 37.7 hours on the Internet per week. When using the Internet for academic tasks, searching information online was reported to be the most frequent activity. The logs of four participants were unavailable/incomplete due to their failure to follow the instructions to log in. Thus only 8 logs were included. We recorded their search activities with SCOOP as they searched for answers to two essay questions: How do bees choose where to build their new homes? And what are the implications for human life? Both the task questions and students’ answers were presented in Chinese. The students were allowed to finish the task in their own paces. They were also encouraged to highlight the information they found relevant to the task by using the highlight function in SCOOP. The writing task performance was scored between 0 and 10 based on the accuracy and depth of the answers. We evaluated the data by redrawing the search path for each participant and then visually comparing the patterns across participants. In this study we analyzed general trends and identified recognizable patterns. The rest of this section details the most prominent features found in the data set.
Upon observing students’ mouse behavior during the search, we classified their search behavior into five categories: search, read information, review task questions, highlight relevant information, and compose answers. These five activities constitute the search path for each participant. There was no instance wherein participants could locate an answer with only one attempt. In other words, they needed to adapt their search strategies when they were unsuccessful during their initial attempts. To better capture this regulatory process, pattern-based analysis was conducted to describe the sequence of activities performed during a specific period of time and presented in a time-based diagram. Thus, a time-series sequence chart was created for each participant based on his log.
We characterized the participants into four types of searcher. Participant 1, 2, and 6 were “active regulatory searchers” who typically devoted the first stage of their search to “search by reviewing the task questions”. This was followed by “read information and compose answers”. The search process was ended by “search more info � read � add to answers”. Participant 12 represented an “inactive regulatory searcher” who followed a similar pattern yet spent significantly less time on all types of activities. The frequency of updating search queries was much lower, and the composition of answers did not start until the middle of the search process. This might explain the difference in their performance levels. Participant 1, 2, and 6 scored highest among the participants while Participant 12 scored lowest. It appears that regulating the process by referring back to the task question to ensure the search was on the right track and looking for most effective search queries led to better task performance. Sufficient engagement in these regulatory activities is a basic requirement.
The rest participants approached the task in different ways. Participant 9 and 11 were eager to work on the task, indicated by their “search-read-answer” behavior at the beginning. This pattern was repeated until the task was finished. Both of them were observed not to review the task questions at all. Not surprisingly, this lack of monitoring of the search process resulted in poor performance. They were classified as “non- regulatory searchers”. Following a similar pattern, however, participant 7 accomplished the task efficiently and successfully. We speculated that this student might have a better prior knowledge of the topic which compensated the lack of monitoring or high ability of choosing effective queries. Participant 4 did review the task questions quite a few times in the initial stage, yet this participant failed to judge the relevance of the information, which did not bring out good performance. He was classified as an “incapable searcher”. Figure 3 presented the search patterns of selected participants.
Conclusion
We developed an online tool for tracking mouse behavior on web in order to build a more extensive account of student web search process. We conducted a user study to analyze these behaviors and investigate behavior trends. We found that when users regulate their search process more often (by reviewing the task requirement or updating the search query), the task performance is better. Yet the regulatory activities require a decent level of dedication and the ability of differentiating relevant from irrelevant information also counts for task performance to some degree. Future studies are warranted to investigate further how to prompt users to regulate their search processand certain interventions can be designed accordingly.
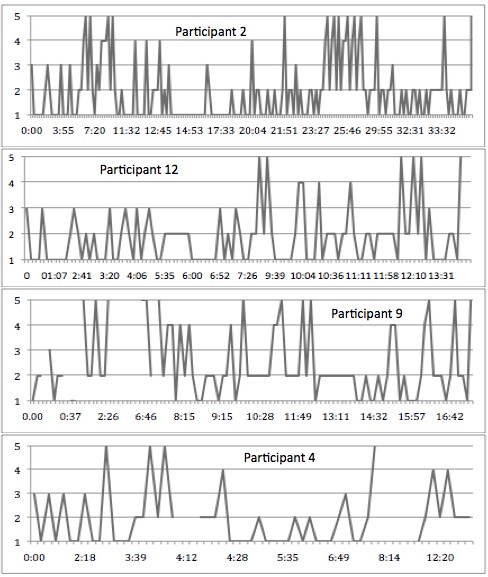
Acknowledgment
This research was supported by the Youth Scholar Grant from Sun Yat-sen University awarded to Yabo Xu (62000-3161032 and 62000-3165002). The authors declare that there is no conflict of interest.
References
Freeman, J. B., & Ambady, N. (2010). MouseTracker: Software for studying real-time mental processing using a computer mouse-tracking method. Behavior Research Methods, 42, 226-241.
Goecks J., & Shavlik J. (2000). Learning users’ interests by unobtrusively observing their normal behavior. In Proceedings of 2000 Inernational Conference on Intelligent User Interfaces (pp. 129-132). New York, NY: ACM Press.
Hirsch, S. G. (1999). Children’s relevance criteria and information seeking on electronic resources. Journal of the American Society for Information Science, 50, 1265-1283. https://doi.org/10.1002/(SICI)1097-4571(1999)50:14<1265::AID-ASI2>3.0.CO;2-E
Hong, J. I. Heer, J., Waterson, S., & Landay J. A. (2001). WebQuilt: A framework for capturing and visualizing the Web experience. ACM Transactions on Information Systems, 19, 263-285.
Jansen, B. J. (2005). Evaluating success in search systems. In Proceedings of the 66th Annual Meeting of the American Society for Information Science & Technology (Vol. 42). Charlotte, North Carolina.
MaKinster, J. G., Beghetto, R. A., & Plucker, J. A. (2002). Why can’t I find Newton’s third law? Case studies of students’ use of the Web as a science resource. Journal of Science Education and Technology, 11, 155–172.
Monereo, C., Fuentes, M., & Sànchez, S. (2000). Internet search and navigation strategies used by experts and beginners. Interactive Educational Multimedia, 1, 24-34.
Mueller, F., & Lockerd, A. (2001). Cheese: Tracking mouse movement activity on websites, a tool for user modeling. In Proceedings of the CHI '01 extended abstracts on Human factors in computing systems (pp. 279-280). New York, NY: Association for Computing Machinery.
Reeder, R. W., Pirolli, P., & Card, S. K. (2000). WebLogger: A data collection tool for Web-use studies. Xerox PARC, Technical Report.
Turnbull, D. (2006). Methodologies for understanding Web use with logging in context.
Copyright information
This work is licensed under a Creative Commons Attribution-NonCommercial-NoDerivatives 4.0 International License.