Abstract
There is a growing body of evidence showing that personal epistemology is a critical component of student learning (Hofer, 2001). Developed by Schraw, Dunkle, and Bendixen (1995), and based on the earlier work on Schommer (1990), the Epistemic Beliefs Inventory (EBI) was designed to measure five constructs concerning the nature of knowledge and the origins of individuals’ abilities. The primary purpose of this study was to reevaluate the psychometric properties of the EBI as it continues to be used in the measurement of epistemic beliefs in a variety of educational and professional settings. Based upon the results of this study, we confirm previous research confirming the lack of stability of the EBI. In addition, a revised structure appears to be present in which only twenty-nine items of the thirty-two items of the EBI are retained. The resulting instrument contains five constructs, likely representing five independent dimensions of epistemic beliefs, although additional research needs to be conducted on this revised model.
Keywords: Epistemic Beliefs Inventory, psychometric tests
Introduction
Epistemology focuses on the nature of knowledge and the justification of belief in one’s knowledge. Arner (1972) divided epistemology into three areas of inquiry: the limits of human knowledge, the sources of human knowledge, and the nature of human knowledge. Inquiry into the limits of human knowledge explores whether there are questions in which it is impossible for humans to be able to acquire evidence so as to be able to rationalize an answer. Inquiry as to the source of human knowledge explores whether sources of knowledge are obtained from experience or from intellectual reason. Examination into the nature of human knowledge analyses the concepts that are prominent in discussions of knowledge. The justification of what one believes and what gives one justification in that belief are central to the nature of justification itself (Arner, 1972; Muis, 2004).
According to Schommer (1990), there are three dimensions of knowledge: certainty of knowledge, source of knowledge, and structure of knowledge. She developed additional dimensions relating to knowledge acquisition: control of knowledge acquisition and speed of knowledge acquisition. Schommer (1994) later proposed that students with simple epistemological beliefs viewed knowledge as finite and believed that knowledge was established at birth, where as those with more sophisticated epistemological beliefs embraced knowledge as complex and asserted that the “source of knowledge shifts from the simple transfer of knowledge from authority to process of rational thinking” (p. 295).
There is a growing body of evidence showing that personal epistemology is a critical component of student learning (Hofer, 2001). For nearly fifty years, studies have been conducted on the subject of epistemological beliefs with the hope of establishing a better understanding of relationship between knowledge and learning (e.g., Perry, 1968; Dweck & Leggett, 1988; Hammer, 1994; Hoffer & Pintrich, 1997, 2002; Magno, 2011; Schommer & Walker, 1997; Schraw, Dunkle, & Bendixen, 1995, Ren, Baker, & Zhang, 2009). Magno (2010) supported the findings of Schwartz and Bardi (2001) by showing that Asian values of education were reflected in their epistemic beliefs about learning. Most recently, Teo and Chai (2011) confirmed previous studies which indicated the instability of the Epistemic Beliefs Inventory (EBI) and called for researchers to continue to re-visit the instruments psychometric properties.
As a result of this relationship, numerous instruments have been developed to collect data regarding individuals’ beliefs about the nature of knowledge. Such instruments include the Checklist of Educational Views (Perry, 1968), the Epistemic Doubt Interview (Boyes & Chandler, 1992), the Attitudes Toward Thinking and Learning Survey (Galotti, Clinchy, Ainsworth, Lavin, & Mansfield, 1999), the Schommer Epistemological Questionnaire (Schommer, 1990), and the Epistemic Beliefs Inventory (Schraw et al., 1995).
The aforementioned research demonstrates an interest in the role of epistemological beliefs on learning, and thus a need to measure epistemological beliefs. Developed by Schraw, Dunkle, and Bendixen (1995), and based on the earlier work on Schommer (1990), the Epistemic Beliefs Inventory (EBI) was designed to measure five constructs concerning the nature of knowledge and the origins of individuals’ abilities. Certain Knowledge concerns whether absolute knowledge exists or does it change over time. Innate Ability explores whether the ability to acquire knowledge is endowed at birth. Quick Learning examines whether learning occurs in a quick or not-at-all fashion. Simple Knowledge focuses on whether knowledge consists of discrete facts. Finally, Omniscient Authority indicates whether knowledge is transmitted by authorities or obtained through personal experience (Nietfeld & Enders, 2003).
Problem Statement
Research examining the EBI has produced inconsistent findings, however. Nussbaum and Bendixen (2003) were unsuccessful in reproducing the EBI’s five-factor structure. In their initial study, exploratory factor analysis produced only two factors: Complexity, which included items designed to measure innate ability, simple knowledge, and quick learning, and Uncertainty, which included factors designed to measure certain knowledge and omniscient authority. In the following year, Nussbaum and Bendixen’s analysis of the EBI produced three factors: Simple Knowledge, Certain Knowledge, and Innate Ability (2003). Müller, Rebmann, and Liebsch (2008) identified a four-factor structure in the EBI: Speed of Knowledge Acquisition, Control of Learning Processes, Source of Knowledge, and Structure/Certainty of Knowledge. In a cross- cultural pilot-study in Germany and Australia, Sulimma (2009) was only able to identify three factors in the EBI: Structure, Source, and Control. Laster (2010) was able to identify four factors: Innate Ability, Quick and Certain Knowledge, Simple Knowledge, and Source of Absolute Knowledge.
Other recent studies also have shown similar inconsistencies with the reliability of the EBI. Ravindran et al. (2005) reported Cronbach’s alpha coefficients for the five subscales ranging from .54 to .78 while DeBacker, Crowson, Beesley, Thoma, and Hestevold (2008) also reported coefficients below .70 in all five subscales. These results are disconcerting and indicate difficulty in the operationalization of the constructs underlying epistemic beliefs. Table 1 provides a comparison of these findings.
Most recently, Teo and Chai (2011) were unsuccessful in their attempt to replicate the five- factor model of the EBI (Schraw et al., 1995). Using a sample of over 1,800 teachers from Singapore, they reported that Schraw’s five-factor model did not fit values such as CFI, TLI, RMSEA, and SRMR. Teo and Chai state that additional research needs to be conducted on the EBI to further understand what items in the EBI are applicable in different cultures.
Purpose of the Study
Because of the psychometric concerns of the EBI, the instrument’s developers have urged researchers to continue examining the construct validity of the EBI, noting that one of the main challenges for researchers studying epistemic beliefs has been the lack of valid and reliable self- reporting instruments (Bendixen, Schraw, & Dunkle, 1998; Schraw, Bendixen, and Dunkle, 2002). Therefore, the purpose of this study is to re-evaluate the psychometric properties of the EBI as it continues to be used in the measurement of epistemic beliefs in a variety of educational and professional settings.
Analytical Considerations
While it is evident that the reliability and validity of the EBI are unconfirmed, it continues to be used in the measurement of epistemic beliefs in a variety of educational and professional settings. With the growing interest in epistemic beliefs, it is imperative that researchers have valid and reliable instruments. Therefore, the current study sought to explore whether the current authors could improve the EBI by conducting an in-depth analysis of the five original scales and exploring other scale structures.
According to Sass (2010), either exploratory or confirmatory factor analysis procedures may be used to test the expected structure of an instrument. In its purest form, exploratory factor analysis (EFA) serves to determine, through statistical exploration, the underlying constructs that influence responses to a given set of items. EFA is used when the researcher lacks clear a priori evidence about the number of factors, and is instead intending to generate theory (Stevens, 2009). Confirmatory factor analysis (CFA), on the other hand, seeks to establish the validity of a model through the calculation of statistical measures of model fit to determine whether the underlying constructs influence the responses in the expected manner (Nunnally, 1978). In this way, CFA is a theory-testing procedure (Stevens, 2009).
When utilizing either exploratory or confirmatory factor analysis, however, numerous decisions must be made to ensure the stability of the factor structure and interpretation (Sass, 2010). As with all statistical procedures, researchers must consider what sample size would be appropriate for the analyses in question. This determination is problematic for factor analyses, however, as there is wide variation in the expected requirements. As indicated by de Winter and his colleagues (2009), some researchers emphasize an absolute number (i.e., 50, 300, 1000), while others emphasize a participant to item ratio (e.g., 5:1, 10:1, 20:1). Recent studies have indicated that sample size requirements vary according to observed communalities, strength of factor loadings, the number of variables per factor, and the number of extracted factors (2009).
Despite these sampling concerns, and lack of agreement regarding necessary sample sizes, many of the existing studies on the EBI have utilized relatively samples that fail to most of these guidelines, and could result in the unreliability of the results as presented above.
Other considerations impacting the stability of the factor structure are the model fitting and estimation procedures used (Flora & Curran, 2004), the choice of the number of factors to extract (Horn, 1965), the method of factor extraction (Hayton, Allen, & Scarpello, 2004), the correlation matrix (Jöreskog & Moustaki, 2001), and the rotation method (Browne, 2001). According to MacCallum and Tucker (1991), factor recovery improves through the increase emphasis on any of the above components. Failure to adequately consider each of these decision points may result in a factor structure that lacks sufficient validity and is thus unable to be replicated (Crocker & Algina, 2006).
Research Methods
Participants
A sample of 282 undergraduate students in an environmental science class at a medium sized research university in the Midwestern United States participated in this study at the beginning of the fall semester. The demographic distribution of the students responding to the survey is listed in Table 2.
Over 91% of the participants recorded “Caucasian (non-Hispanic)” as their ethnicity. Of the remaining students, 0.4% identified themselves as American Indian, 1.8% as Asian, 1.8% as Black, 0.7% as Hispanic/Latino, and 3.0% responded with “rather not state.” These demographics are consistent with recent undergraduate statistics for this university over the last several years.
Instrumentation
The primary instrument used in this study was Schraw, Dunkle, and Bendixen’s (1995) Epistemic Belief Inventory. The EBI consists of 32 statements for which individuals respond using a 5-point Likert-type rating scale from strongly disagree (1) to strongly agree (5) to items concerning their beliefs about education and learning. As previously noted, the inventory was developed to measure five underlying constructs: Certain Knowledge, Innate Ability, Quick Learning, Simple Knowledge, and Omniscient Authority. Additional items included demographic questions such as age, sex, race/ethnicity, major, and academic classification.
Procedure
The EBI was administered via Survey Monkey during the first two weeks of the fall semester. Upon providing consent to participate in the electronic survey, students were directed to respond to the 32 items on the Epistemic Beliefs Inventory and then to complete a variety of demographic items as described above. Completing the survey was not part of the class requirements and no additional credit was given to students who completed the survey.
Data Analysis
An initial maximum-likelihood confirmatory factor analysis was conducted using LISREL 8.80 software (Jöreskog & Sörbom, 2006) to evaluate whether there was evidence for the five- factor structure proposed by Bendixen, Schraw, and Dunkle (1998). Specifically, the original model consisting of eight items representing Simple Knowledge, seven items representing Certain Knowledge, five items representing Omniscient Authority, five items representing Quick Learning, and seven items representing Innate Ability was tested for adequate model fit. Numerous fit indices were considered simultaneously to evaluate model fit, including the observed chi-square values, the normed fit index (NFI), the non-normed fit index (NNFI), the comparative fit index (CFI), the goodness of fit index (GFI), the standardized root mean-squared residual (SRMR), and the root mean square error of approximation (RMSEA). For this study, a combination of χ2/df ratio less than 3, GFI greater than 0.95, NFI, NNFI, CFI, and GFI greater than .90, SRMR less than 0.08, and RMSEA less than .06 was considered to be satisfactory (Hu & Bentler, 1999). While it is possible for some indices to indicate fit while others will indicate a slight lack of fit due to the differing mathematical underpinnings of each index, the majority of the indices were expected to meet the established criteria while the remaining indices were expected to be close to these criteria (Marsh, Hau, & Wen, 2004).
Results
As all surveys were answered completely, no procedures to account for missing data were necessary, and because data were collected online, no responses existed outside the expected range. To assess the assumption of univariate normality, a necessary condition for maximum likelihood analysis, skewness and kurtosis of each item was examined. According to Kline (2011), skewness less than |3| and kurtosis less than |8| indicate minimal concerns with univariate normality. For this study, the skewness ranged from -1.027 to 1.038 and the kurtosis ranged from -1.011 to 2.664, indicating that the responses were sufficiently normally distributed. The means, standard deviations, skewness, and kurtosis for each of the 32 indicators are presented in Table 3.
Confirmatory Analysis
An initial maximum-likelihood confirmatory factor analysis was conducted using LISREL 8.80 software (Jöreskog & Sörbom, 2006) to evaluate whether there was evidence for the five- factor structure proposed by Bendixen, Schraw, and Dunkle (1998). To accomplish this, the raw data was used to construct The observed chi-square value and degrees of freedom, four goodness-of-fit indices, and two misfit measures for the original model were consistently unsatisfactory: χ2 (105) = 412.02, p<.001; χ2/df ratio = 3.92; GFI = .84, NFI=.46, NNFI=.45, CFI= .52; SRMR = .10; and RMSEA=.08. These results clearly indicated that there was a considerable degree of misfit between the original five-factor model and these data.
Exploratory Analyses
As the results of the confirmatory analyses failed to support the EBI as originally specified, and because other studies examining the EBI also fail to reach consensus concerning the underlying latent structure of the instrument, further analysis was deemed necessary. Initial efforts exploring the modification indices and the standardized residual matrix (Schumacker & Lomax, 2010) indicated that substantial revisions would be necessary to modify the model to an acceptable fit. Proceeding in such a fashion results in dangers concerning data-driven modification and lack of theoretical justification for the changes (Kelloway, 1998), which were deemed too risky for this study. Instead, Brown (2006) suggests that when such decisions cannot be directly supported given existing information such as prior research, it becomes appropriate to return to exploratory analyses.
Due to the relative ease of conducting EFA procedures as compared to CFA procedures, and given the large degree of misfit found in the current CFA analysis as well as the existing literature, exploratory analysis was used to determine the underlying structure for the 32 items on the EBI. Thus, additional exploratory analyses were conducted using PASW Statistics 18 (SPSS, 2010) to further examine the underlying structure of the EBI. The choice to utilize exploratory methods was considered appropriate as exploratory factor analysis should be used to serve as an initial test of the latent structure underlying items on an instrument (Stapleton, 1997).
A principal component analysis (PCA) was conducted on the 32 items with orthogonal rotation (Varimax) using SPSS. The Kaiser-Meyer-Olkin statistic indicated that the overall sampling adequacy was good (KMO = .70), and all KMO values were at least .57, which is above the generally acceptable cut-off of .50 (Kaiser, 1974), indicating that it was appropriate to perform factor analysis. Bartlett’s test of sphericity also indicated that correlations between items were sufficiently large for the analysis (χ2 (496) = 1966.27, p < .001). Initial results produced ten components with eigenvalues above Kaiser’s (1960) criterion of 1.00, which together explained 58.69% of the variance. The scree plot was somewhat ambiguous, though generally supported eight components suitable for extraction (Figure 1).
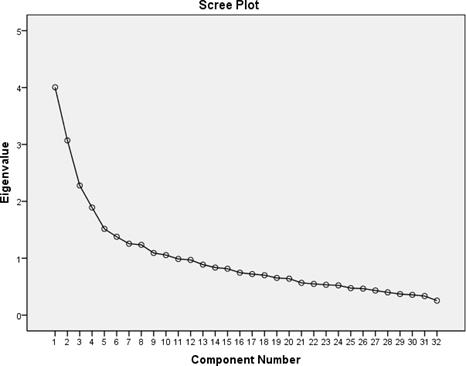
Given the relative ambiguity of scree plots and the known overestimation of results associated with both scree plots and Kaiser’s (1960) rule, further analysis was necessary to determine the resulting number of components. Parallel analysis (Horn, 1965) was used to further clarify the number of underlying constructs for the 32 items on the EBI. Parallel analysis is an empirical method used to determine the number of underlying constructs that create the variance in a set of items and indicate the number of factors or components that should thus be retained (1965). This is accomplished by comparing the observed eigenvalues against the eigenvalues that would be expected to occur at random. For this study, parallel analysis identified five underlying constructs, or five potential components to be extracted. Figure 2 provides a graphical output of the results.
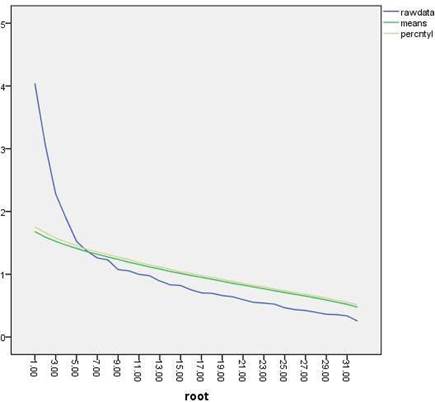
While Kaiser’s rule and the scree plot resulted in inconclusive results, parallel analysis provided evidence supporting a five-factor structure, albeit a different structure than that proposed by Schraw, Dunkle, and Bendixen (1995). Thus, five factors were extracted, resulting in simple structure with all but one item loading on one and only one component and all factors consisting of at least four items. Table 5 shows the factor loadings after Varimax rotation.
A closer examination of the non-loading item revealed that it contained a “double-barreled” statement: “I like teachers who present several competing theories and let their students decide which is best.” This creates a potential problem in that it is impossible for the researchers to know which part of the questions was answered.
While this resulting structure possesses the same number of factors as the model proposed by Schraw, Dunkle, and Bendixen (1995), the constructs underlying the structure appear to be different given that the items load in a different manner than expected according to the original model. Upon determining that this five-factor structure was the best fit for the data, additional reliability analyses were performed to provide a more consistent instrument that is also more easily interpretable. During these reliability analyses, two additional items were removed. Table 6 shows the five resulting factors with the number of items and scale descriptive statistics including item means, standard deviations, and Cronbach’s alpha reliability.
Overall, these analyses indicated that five distinct constructs were underlying participants’ responses to the EBI items, though these factors differed in terms of internal consistency even after excluding items to improve alpha levels as much as possible. Specifically, three of the five scales resulting scales had acceptable consistency, one possessed questionable consistency, while the final scale possessed unacceptable consistency (George & Mallery, 2003).
Discussion
After conducting tests of internal consistency and several different exploratory factor analyses, the multidimensionality of the instrument was confirmed. However, we were unable to replicate the reported structure of the EBI as constructed by Schraw, Dunkle, and Bendixen (1995) nor did any of the structures previously found (Schommer, 1990; Nussbaum and Bendixen, 2003; Müller, Rebmann, and Liebsch, 2008; Sulimma, 2009) emerge. The factor identified by Schraw (1995) as omniscient authority (Q4, Q7, Q20, Q27, Q28) did not emerge from our analysis. Kardas and Wood (2000) were also unable to isolate Omniscient Authority, the beliefs in the source of knowledge, as a unique factor. Much as Teo & Chai (2011) found, we do not have an interpretable solution and any attempt to interpret a solution would be inappropriate. This study, as well the previously mentioned studies, should serve as a warning to researchers using this instrument in its current form.
Based upon the results of this study, only twenty-nine items of the thirty-two items of the EBI were retained. The resulting instrument, presented in Table 7, contains five factors, representing five independent dimensions of epistemic beliefs.
Four of the five identified factors are closely related to those identified by Schommer (1990, 1994) and Schraw, Bendixen, Dunkle (2002). Factor 1 corresponds to items related to the belief of knowledge as either dualistic or relative. Factor 2 includes items pertaining to the belief that learning is simple or complex. Factor 3 contains items which identify the belief of learning as either being perceived from an incremental or entity perspective. Factor 4 alludes to the idea of the presence of truth. Factor 5 is not similar to that those described by Schommer (1990, 1994) or Schraw, Bendixen, Dunkle (2002). The items in Factor 5 convey the belief that there is a predetermined amount of time necessary for learning. Additional research needs to be conducted on this factor to further isolate and identify the relationship within the items.
- Limitations and Future Research
The results of this study must be interpreted with extreme caution as two of the five scales were found to have questionable or unacceptable internal consistency, indicating additional problems that were not identified during the component analysis. While the component analysis resulted in simple structure with the five components identified through parallel analysis, the lack of consistency of two scales, coupled with the knowledge that internal consistency was not particularly good for any of the scales, indicates probable concerns with both the items and the operationalization of the epistemological constructs. This is not overly surprising given the differing results in the aforementioned literature, Further, recent research by Teo and Chai (2011) resulted in an inability by these researchers to produce any interpretable solutions, causing further concern.
Several additional limitations may warrant consideration. First, while the sample size is adequate according to most standards for factor and principle component analysis, there is disagreement to the specific requirements as mentioned previously. It is conceivable that sampling bias could have resulted in the current sample, which could have resulted in either too many or two few factors (Costello & Osborne, 2005). According to Costello and Osborne, an insufficient sample could impact the resulting structure by changing the number of factors or even resulting in items being misclassified as belonging to the incorrect component.
Second, while the authors believe that the participants in this study do not differ meaningfully from the intended population for the Epistemic Beliefs Inventory, the fact that a convenience sample was sought in environmental science courses at a mid-size Midwestern university could pose another limitation. Specifically, the nature of students enrolled in such courses could indeed result in differences that are unknown to the researchers, and the geographic region may also have an impact upon the results.
Lastly, the revised structure of the EBI proposed in this study needs additional evaluation and validation. The psychometric instability of previous models is evidence of the need to continuously re-evaluate and refine instruments. Until researchers are able to consistently replicate the structure of the EBI, however, it is recommended that any research conducted using the EBI to explore the relationship between epistemic beliefs and learning be interpreted with extreme caution. To more accurately explore this relationship, more work needs to be done to either validate the current version of EBI as proposed by Schraw, Dunkle, and Bendixen (1995), or to take a step backward by revising the individual items on the EBI to better align with the dimensions proposed in the initial model or in one of the several models that have resulted from analyses. Any effort to revise the items on the EBI, however, should be done in a manner that is well-supported by the theory and literature surrounding epistemic beliefs.
Acknowledgements
The authors declare that there is no conflict of interest.
References
Arner, D. G. (1972). Perception, reason, and knowledge: An introduction to epistemology. Glenview, IL: Scott, Foresman.
Bendixen, L. D., Schraw, G., & Dunkle, M. E. (1998). Epistemic beliefs and moral reasoning. The Journal of Psychology, 132, 187-200. DOI:
Boyes, M. C., & Chandler, M. (1992). Cognitive development, epistemic doubt, and identity formation in adolescence. Journal of Youth Adolescence, 21, 277-303. DOI:
Brown, T. A. (2006). Confirmatory Factor Analysis for Applied Research. New York, NY: Guilford Press.
Browne, M. W. (2001). An overview of analytic rotation in exploratory factor analysis. Multivariate Behavioral Research, 36, 111-150. DOI:
Costello, A. B., & Osborne, J. W. (2005). Best practices in exploratory factor analysis: Four recommendations for getting the most from your analysis. Practical Assessment, Research, & Evaluation, 10(7). http://pareonline.net/getvn.asp?v=10&n=7
Crocker, L., & Algina, J. (2006). Introduction to classical and modern test theory. Pacific Grove, CA: Wadsworth Publishing Company.
DeBacker, T. K., Crowson, H. M., Beesley, A. D., Thoma, S. J., & Hestevold, N. L. (2008). The challenge of measuring epistemic beliefs: An analysis of three self-report instruments. The Journal of Experimental Education, 76(3), 281-312. DOI:
de Winter, J. C. F., Dodou, D., & Wieringa, P. A. (2009). Exploratory factor analysis with small sample sizes. Multivariate Behavioral Research, 44, 147-181. DOI:
Dweck, C. S., & Leggett, E. L. (1988). A social-cognitive approach to motivation and personality. Psychological Review, 95, 239-256. DOI:
Flora, D. B., & Curran P. J. (2004). An empirical evaluation of alternative methods of estimation for confirmatory factor analysis with ordinal data. Psychological Methods, 9, 466-491. DOI:
Galotti, K. M., Clinchy, B. M., Ainsworth, K. H., Lavin, B., & Mansfield, A. F. (1999). A new way of assessing ways of knowing: The Attitudes Toward Thinking and Learning Survey (ATTLS). Sex Roles, 40, 745-766.
George, D., & Mallery, P. (2003). SPSS for Windows step by step: A simple guide and reference. 11.0 update (4th ed.). Boston, MA: Allyn & Bacon.
Hammer, D. (1994) Epistemological beliefs in introductory physics. Cognit. Instr., 12, 151-183. DOI:
Hayton, J. C., Allen, D. G., & Scarpello, V. (2004). Factor retention decisions in exploratory factor analysis: A tutorial on parallel analysis. Organizational Research Methods, 7, 191- 205. DOI:
Hofer, B. K. (2001) Personal epistemology research: Implications for learning and teaching. Journal of Educational Psychology Review, 13(4), 353-383.
Hofer, B. K., & Pintrich, P. R. (1997). The development of epistemological theories: Beliefs about knowledge and knowing and their relation to learning. Rev. Educ. Res. 67, 88-140. DOI:
Hofer, B. K., & Pintrich, P. R. (Eds.). (2002). Personal epistemology: The psychology of beliefs about knowledge and knowing. Mahwah, New Jersey; Lawrence Erlbaum Associates. DOI:
Horn, J. L. (1965). A rationale and test for the number of factors in factor analysis. Psychometrika, 30, 179-185. DOI:
Hu, L., & Bentler, R. M. (1999). Cut-off criteria for fit indexes in covariance structure analysis: Conventional criteria versus new alternatives, Structural Equation Modeling, 6(1), 1-55. DOI:
Jöreskog, K. G., & Moustaki, I. (2001). Factor analysis for ordinal variables: A comparison of three approaches. Multivariate Behavioral Research, 36, 347-387. DOI:
Jöreskog, K. G. & Sörbom, D. (2006). LISREL 8.8 for Windows [Computer Software]. Lincolnwood, IL: Scientific Software International, Inc.
Kaiser, H. F. (1960). The Application of Electronic Computers to Factor Analysis. Educational and Psychological Measurement, 20, 141-151. DOI:
Kaiser, H. F. (1974). An index of factorial simplicity. Psychometrika, 39, 31-36. DOI: 10.1007/BF02291575
Kardash, C.M., & Wood, P. (2000, April). An individual item factoring of epistemological beliefs as measured by self-reporting surveys. Paper presented at the American Educational Research Association, New Orleans, LA.
Kelloway, E. K. (1998). Using LISREL for Structural Equation Modeling: A Researcher’s Guide. Thousand Oaks, CA: Sage Publications, Inc.
Kline, R. B. (2011). Principles and Practice of Structural Equation Modeling (3rd Ed.). New York, NY: Guilford Press.
Laster, B. B. (2010). A structural and correlations analysis of two common measures of personal epistemology. (Doctoral dissertation). Retrieved from UMI Dissertation Publishing. UMI Number: 3443573.
MacCallum, R. C., & Tucker, L. R. (1991). Representing sources of error in the common factor model: Implications for theory and practice. Psychological Bulletin, 109, 502–511. DOI:
Magno, C. (2010). Looking at Filipino pre-service teachers’ value for education through epistemological beliefs about learning and Asian values. The Asia-Pacific Education Researcher, 19(1), 67-78. DOI:
Magno, C. (2011). Exploring the relationship between Epistemological Beliefs and self- determination. The International Journal of Research and Review, 7(1), 1-23.
Marsh, H. W., Hau, K, & Wen, Z. (2004) In Search of Golden Rules: Comment on Hypothesis- Testing Approaches to Setting Cut-off Values for Fit Indexes and Dangers in Overgeneralizing Hu and Bentler's (1999) Findings. Structural Equation Modeling: A Multidisciplinary Journal, 11(3), 320-341. DOI:
Müller, S., Rebmann, K., & Liebsch, E. (2008). Trainers’ beliefs about knowledge and learning – A pilot study. European Journal of vocational Training, 45(3), 90-108.
Muis, K. R. (2004). Personal epistemology and mathematics: A critical review and synthesis of research. Review of Educational Research, 74(3), 317-377. DOI:
Nietfeld, J. L., & Enders, C. K. (2003). An examination of student teacher beliefs: Interrelationships between hope, self-efficacy, goal-orientations, and beliefs about learning. Current Issues in Education [On-line], 6(5). Retrieved from http://cie.ed.asu.edu/volume6/number5/
Nunnally, J. C. (1978). Psychometric theory. New York: McGraw-Hill.
Nussbaum, E. M., & Bendixen, L. D. (2003). Approaching and avoiding arguments: The role of epistemological beliefs, need for cognition, and extraverted personality traits. Contemporary Educational Psychology, 28, 573-595. DOI:
Perry, W. G. Jr. (1968). Patterns of development in thought and values of students in a liberal arts college: A validation of a scheme. Final Report. Retrieved from ERIC database.
Ravindran, B., Greene, B. A., & DeBacker, T. K. (2005). The role of achievement goals and epistemological beliefs in the prediction of pre-service teachers’ cognitive engagement and learning. Journal of Educational Research, 98(4), 222-233. DOI:
Ren, Z., Baker, P., & Zhang, S. (2009). Effects of student-written wiki-based textbooks on pre- service teachers’ epistemological beliefs. Journal of Educational Computing Research, 40(4), 429-449. DOI:
Sass, D. A. (2010). Factor loading estimation error and stability using exploratory factor analysis. Educational and Psychological Measurement, 70, 557-577. DOI:
Schommer, M. (1990). Effects of beliefs about the nature of knowledge on comprehension. Journal of Educational Psychology, 82(3), 498-504. DOI:
Schommer, M. (1994). Synthesizing epistemological belief research: Tentative understandings and provocative confusions. Educational Psychology Review, 6(4), 293-319. DOI:
Schommer, M., & Walker, K. (1997). Epistemological beliefs and valuing school: Considerations for college admissions and retention. Research in Higher Education, 38, 173-186. DOI:
Schraw, G., Dunkle, M. E., & Bendixen, L. D. (1995). Cognitive processes in well-defined and ill-defined problem solving. Applied Cognitive Psychology, 9, 523-538. DOI:
Schraw, G., Bendixen, L. D., & Dunkle, M. E. (2002). Development and validation of the Epistemic Belief Inventory (EBI). In B. K. Hofer, & P. R. Pintrich (Eds.), Personal epistemology: The psychology of beliefs about knowledge and knowing (pp. 261-275). Mahwah, NJ: Erlbaum. DOI:
Schumacker R. E. & Lomax, R. G. (2010). A Beginners Guide to Structural Equation Modeling (3rd Ed.). New York, NY: Routledge.
Schwartz, S. H., & Bardi, A. (2001). Value hierarchies across cultures: Taking a similarities perspective. Journal of Cross-cultural Psychology, 32(3), 268-290. DOI:
SPSS. (2010). PASW Statistics Base 18 [Computer Software]. Chicago, IL: SPSS, Inc.
Stapleton, C. D. (1997, January). Basic concepts in exploratory factor analysis (EFA) as a tool to evaluate score validity: A right-brained approach. Paper presented at the meeting of the Southwest Educational Research Association, Austin, TX.
Stevens, J. P. (2009). Applied Multivariate Statistics for the Social Sciences (5th Ed). New York: Taylor and Francis Group.
Sulimma, M. (2009). Relations between epistemological beliefs and culture classifications. Multicultural Education & Technology Journal, 3(1), 74-89. DOI:
Teo, T., & Chai, C. S. (2011). Confirmatory factor analysis of the Epistemic Belief Inventory (EBI): A cross-cultural study. The International Journal of Educational and Psychological Assessment, 9(1), 1-13.
Copyright information
This work is licensed under a Creative Commons Attribution-NonCommercial-NoDerivatives 4.0 International License.